機械学習奮闘記④大規模データを用いたモデル予測
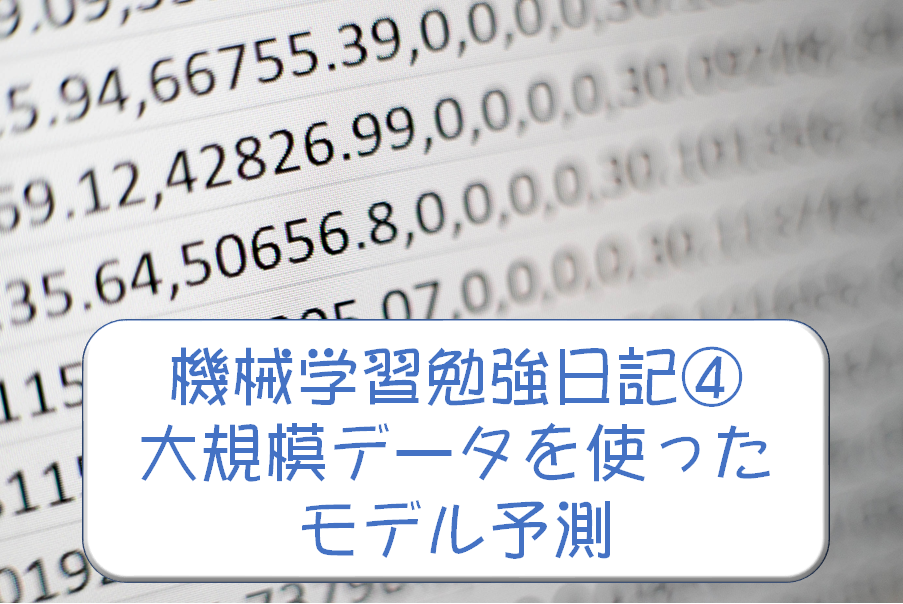
みなさん、こんにちは
シンラボ広報部の福田です。
非テック系の福田による機械学習勉強日記です。
前回はscikit-learnを利用した学習とモデル予測をやってみました。
前回は使っているデータ数が20だけだったので、ちゃんとした解析はできなかったです。今回は「sckit-laern」に付属されているボストンの住宅街のでーを使って、説明変数:平均部屋数(RM)から目的変数:住宅価格(MEDV)を予測するモデルと作ってみました。実際に自分のデータを使うときは、csvファイルを作って、フォルダに入れておけば同様に作業できます。
データの読み込み
最初にpythonを立ち上げます。ここで、
from sklearn.datasets import load_boston
boston = load_boston()
print(boston.DESCR)
と入力して、ボストン住宅街のデータを読み込んでおきます。そうすると下記が表示されます。
.. _boston_dataset: Boston house prices dataset --------------------------- **Data Set Characteristics:** :Number of Instances: 506 :Number of Attributes: 13 numeric/categorical predictive. Median Value (attribute 14) is usually the target. :Attribute Information (in order): - CRIM per capita crime rate by town - ZN proportion of residential land zoned for lots over 25,000 sq.ft. - INDUS proportion of non-retail business acres per town - CHAS Charles River dummy variable (= 1 if tract bounds river; 0 otherwise) - NOX nitric oxides concentration (parts per 10 million) - RM average number of rooms per dwelling - AGE proportion of owner-occupied units built prior to 1940 - DIS weighted distances to five Boston employment centres - RAD index of accessibility to radial highways - TAX full-value property-tax rate per $10,000 - PTRATIO pupil-teacher ratio by town - B 1000(Bk - 0.63)^2 where Bk is the proportion of blacks by town - LSTAT % lower status of the population - MEDV Median value of owner-occupied homes in $1000's :Missing Attribute Values: None :Creator: Harrison, D. and Rubinfeld, D.L. This is a copy of UCI ML housing dataset. https://archive.ics.uci.edu/ml/machine-learning-databases/housing/ This dataset was taken from the StatLib library which is maintained at Carnegie Mellon University. The Boston house-price data of Harrison, D. and Rubinfeld, D.L. 'Hedonic prices and the demand for clean air', J. Environ. Economics & Management, vol.5, 81-102, 1978. Used in Belsley, Kuh & Welsch, 'Regression diagnostics ...', Wiley, 1980. N.B. Various transformations are used in the table on pages 244-261 of the latter. The Boston house-price data has been used in many machine learning papers that address regression problems. .. topic:: References - Belsley, Kuh & Welsch, 'Regression diagnostics: Identifying Influential Data and Sources of Collinearity', Wiley, 1980. 244-261. - Quinlan,R. (1993). Combining Instance-Based and Model-Based Learning. In Proceedings on the Tenth International Conference of Machine Learning, 236-243, University of Massachusetts, Amherst. Morgan Kaufmann.
次に、pandasモジュールの「dataframe」にも同じデータを読み込みます。
import pandas as pd # 必要なデータの読み込み
df=pd.DataFrame(boston.data,columns = boston.feature_names) #説明変数の読み込み
df[‘MEDV’] = boston.target #目的変数の読み込み
x=df.RM.to_frame()
y=df.MEDV
print(x)
print(y)
上記のように入力すると、データが下記のように表示されます。
RM 0 6.575 1 6.421 2 7.185 3 6.998 4 7.147 .. ... 501 6.593 502 6.120 503 6.976 504 6.794 505 6.030 [506 rows x 1 columns] 0 24.0 1 21.6 2 34.7 3 33.4 4 36.2 ... 501 22.4 502 20.6 503 23.9 504 22.0 505 11.9 Name: MEDV, Length: 506, dtype: float64
訓練データと評価データの準備
データの読み込みが終わったので、次に訓練データと評価データを準備します。下記のように入力します。ここで、訓練データの割合は前回同様に30%としています。ここを変更するときは、「size=0.3」の「0.3」の部分を変更します。
from sklearn.model_selection import train_test_split
#訓練データと評価データの準備
(x_train, x_test, y_train, y_test) = train_test_split(x, y, test_size=0.3)
次の段階は機械学習のアルゴリズムを選択します。どういったモデルが最適かは実際のデータと解析結果を見て判断する必要がありますが、ここでは最も単純な線形回帰モデルを使います。
from sklearn.linear_model import LinearRegression
#線形回帰をアルゴリズムとして選択
Ir = LinearRegression()
この後では、訓練データを使って予測モデルを生成します。ここでは、fit()関数を用います。
#モデル学習
lr.fit(x_train, y_train)
予測モデルができたら、評価データを使って予測を行います。ここでは、predict()関数を用います。
#評価データを用いた予測
y_pred=Ir.predict(x_test)
print(y_pred[0]*1000)
最後に予測結果の正確さを評価します。理系の人なら良く出てくる最小二乗法を用います。評価データと予測データの差分の平均二乗誤差を、下記のコマンドを使って計算します。そうすると、最後に平均二乗誤差が表示されます。
from sklearn.metrics import mean_squared_error
# モデルの評価
mse=mean_squared_error(y_test, y_pred)
print(mse)
ここまででビックデータを用いた解析の基本的なことはできるようになりました。この、ボストンのデータは他にも色々とありますので、下記のコマンドで活用してみてください。
・CRIM:人口1人当たりの犯罪発生率
・ZN:25,000平方フィート以上の住居区画の占める割合
・INDUS:小売業以外の商業が占める面積の割合
・CHAS:チャールズ川によるダミー変数(1:川の周辺、0:それ以外)
・NOX:NOx濃度
・RM:住居の平均部屋数
・AGE:1940年より前に建てられた物件の割合
・DIS:5つのボストン市の雇用施設からの距離
・RAD:感情高速道路へのアクセルしやすさ
・TAX:$10,000当たりの不動産税率の総計
・PTRATIO:町ごとの児童と教師の比率
・B:町ごとの黒人(Bk)の比率を次の式で表したもの:1000(BK-0.63)2
・LSTAT:給与の低い職業に従事する人口の割合
今回はビックデータを用いた解析を行ってみました。これで、一通り使いこなせるよな気分になってきました。ただ、目的変数をより精度よく表現する線形モデルを構築するためには、特徴量(説明変数)選択や正則化を行うことを検討する必要があります。その辺の内容は今後の記事でまとめていきます。
EVENTS




